Atlas AI is pioneering in the image data mining front by developing an interactive, real-time Image Data Science toolkit that empowers the user with SQL-like query functionality directly in the image space.
The IDS toolkit uses advanced Graph-theoretic constructs coupled with state-of-the-art Machine Learning algorithms for user-driven image segmentation. Each segment is delivered with a pool of features that describe it in structural, textural and radiometric terms.
These features can be in turn, sourced into existing data-science frameworks for further analytics that offer unique insights into the image information content.
IDS was developed to address segmentation challenges in the absence of training data and suitable or suitably-trained models. It can be operated in three different modes:
- Supervised mode: no prior knowledge on targeted image features,
- Semi-supervised mode: driven by limited-size collections of binary object templates,
- Unsupervised mode: driven by limited-size collections of training data or by prescribed parametrization.
IDS can be used to deliver real-time segmentation output and insights or generate new training data-sets by avoiding the time and cost intensive manual annotation.
The images below demonstrate the use of IDS in construction site monitoring under the supervised segmentation schema, i.e. in the complete absence of training data.
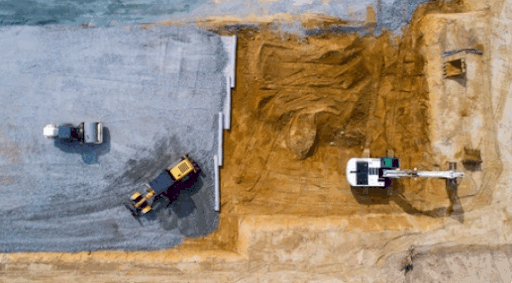

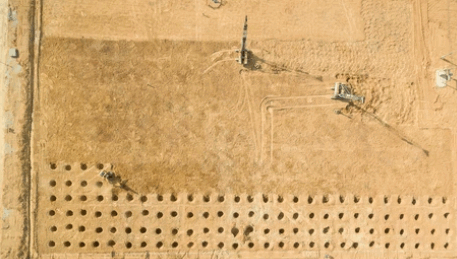



